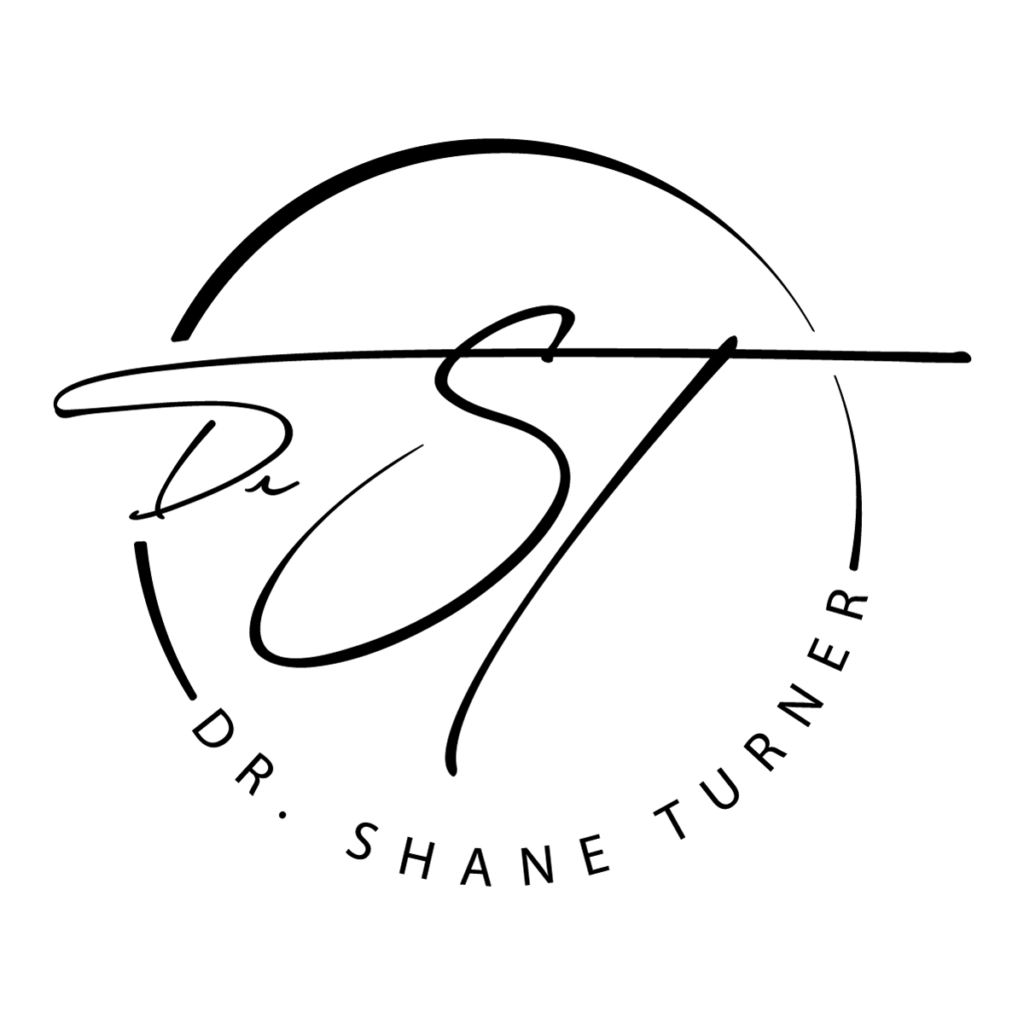
Shane Turner, D.B.A
21 May 2025
Executive Summary
The Department of Defense (DoD) is undergoing a significant transformation through the adoption of Digital Engineering (DE) practices, a strategic imperative driven by the need to accelerate capability delivery and maintain technological superiority in an increasingly complex global landscape. Central to this transformation is the Digital Engineering Ecosystem (DEE), the interconnected infrastructure, environment, and methodologies that enable the effective application of DE. This report provides a detailed analysis of the DEE as it specifically pertains to the DoD Test and Evaluation (T&E) domain. It examines the foundational policies, core components, critical enablers, benefits, challenges, and strategic recommendations for maturing this ecosystem.
The report finds that the T&E DEE is a multifaceted construct involving diverse stakeholders, digitally transformed processes, advanced tools and platforms, robust supporting infrastructure, and governing standards. Key enablers such as the Authoritative Source of Truth (ASoT), digital models, digital twins, the digital thread, Model-Based Systems Engineering (MBSE), and Artificial Intelligence/Machine Learning (AI/ML) are fundamentally reshaping how T&E is planned, executed, and utilized to inform decision-making. While the potential benefits—including improved efficiency, reduced costs, enhanced speed, and better risk management—are substantial, significant technical, cultural, organizational, and workforce challenges must be addressed.
Successfully navigating these challenges and realizing the full potential of the T&E DEE requires a holistic, integrated approach. This includes prioritizing interoperability standards, investing in continuous workforce development, establishing robust governance for data and models, and fostering a culture of collaboration and digital fluency. A mature T&E DEE is not merely a technological upgrade but a critical enabler for the DoD to field superior, resilient, and adaptable capabilities to the warfighter at the speed of relevance, ensuring mission success in the face of evolving threats.
I. The Digital Engineering Ecosystem in the Context of DoD Test & Evaluation
The successful modernization of Department of Defense (DoD) capabilities hinges on a profound shift in engineering practices, with Digital Engineering (DE) at its core. This transformation necessitates a comprehensive understanding of the Digital Engineering Ecosystem (DEE) and its specific application within the critical domain of Test and Evaluation (T&E). The strategic drive towards DE within the DoD, underscored by foundational policies, aims to revolutionize how systems are designed, developed, tested, and sustained, with T&E playing a pivotal role in this new paradigm.
A. Defining Digital Engineering and its Ecosystem for the DoD
Digital Engineering, as embraced by the DoD, signifies an integrated digital approach that utilizes authoritative sources of system data and models as a continuous thread across various disciplines to support all lifecycle activities, from concept through disposal.1 This methodology harnesses the power of computational technology, sophisticated modeling techniques, advanced analytics, and data sciences to modernize and enhance traditional systems engineering practices.3
The Digital Engineering Ecosystem (DEE) is the operational backbone that makes DE feasible. It is defined as the interconnected infrastructure, environment, and methodology—comprising processes, methods, and tools—that are collectively used to store, access, analyze, and visualize the evolving data and models of systems. The primary purpose of this ecosystem is to effectively address the diverse needs of its stakeholders.4 DoD Instruction 5000.97 further refines this by describing the DEE as the necessary infrastructure and architecture, including hardware, software, networks, tools, and the skilled workforce, required to support digital methodologies throughout all phases of a system’s development lifecycle.5 This ecosystem is designed to connect every phase of a system’s lifecycle, thereby ensuring the development and maintenance of technically accurate digital representations of systems and their corresponding digital twins.6
The inherent structure of the DEE, with its emphasis on interconnectedness and comprehensive support mechanisms, directly facilitates the overarching goals of DE. DE’s ambition for an “integrated digital approach” 1 finds its practical realization in the DEE’s provision of “interconnected infrastructure, environment, and methodology”.4 Without this carefully constructed ecosystem, DE practices would likely devolve into a collection of isolated digital activities, failing to achieve the cohesive and synergistic strategy envisioned by the DoD. The core components of the ecosystem—infrastructure, environment, and methodology—are not merely present but are essential preconditions for DE to function effectively and deliver its intended benefits.
Furthermore, the consistent emphasis on “evolving systems’ data and models” 4 within the definition of the DEE carries significant implications for T&E. It necessitates that the T&E DEE be inherently dynamic and highly adaptable. This ecosystem must be capable of seamlessly handling constant updates, modifications, and the continuous influx of new information. This represents a substantial departure from traditional, often static, document-centric T&E approaches. Systems undergoing test and evaluation, particularly those with significant software components or those incorporating AI, evolve at a rapid pace. T&E processes must not only keep pace with this evolution but also be able to assess the impact of these changes effectively. An ecosystem designed to manage “evolving data and models” inherently supports agile T&E methodologies, facilitates continuous integration and continuous testing (CI/CT), and enables the robust evaluation of systems that are designed to change over their operational lifespan—a key strategic pillar identified by the Directorate of Operational Test and Evaluation (DOT&E).7 This dynamic nature contrasts sharply with historical T&E paradigms, which often tested fixed system configurations at discrete, predetermined points in the development cycle.
B. Strategic Imperative: DoD’s Drive Towards Digital Transformation in T&E
The DoD’s adoption of Digital Engineering is not an isolated initiative but a strategic imperative, fundamentally driven by the urgent need for increased speed, agility, and adaptability in response to rapidly evolving global threats and accelerating technological advancements. This imperative is clearly articulated in numerous strategic documents emanating from various branches and levels of the DoD. The 2018 DoD Digital Engineering Strategy, for instance, champions the use of digital representations of systems and components, along with digital artifacts, as the primary means to design, develop, and sustain national defense systems.8 This foundational strategy outlines five crucial goals: formalizing the development and use of models, providing an enduring Authoritative Source of Truth (ASoT), incorporating technological innovation into engineering practices, establishing the necessary supporting infrastructure and environments, and transforming the culture and workforce to embrace digital engineering across the entire lifecycle.9
Digital Engineering is viewed as a “fundamental component” that enables rapid and informed decision-making, facilitates agile acquisition processes, and supports the timely fielding of dominant weapon systems.9 The Department of the Navy similarly regards DE as indispensable for conducting its business effectively in the 21st century.9 More recently, the U.S. Army’s 2024 policy directive calls for the swift adoption of modern DE practices to accelerate its modernization strategy, improve the early identification of cost drivers in system designs, and ultimately deliver needed capabilities to soldiers more quickly.10
This enterprise-wide push directly impacts the T&E community. The DOT&E Strategy Update 2022 explicitly aims for transformative changes across T&E infrastructure, tools, processes, and workforce capabilities. These changes are deemed essential to effectively counter advanced threats and evaluate new technologies, with a core objective to “Accelerate the Delivery of Weapons That Work”.7 Digital Engineering and Modeling and Simulation (M&S) are identified as key enablers that optimize processes, automate aspects of design, development, and integration, and critically, allow for T&E activities to be conducted earlier, more frequently, and with greater thoroughness than traditional approaches permit.3
The consistent emphasis across these strategic pronouncements on terms such as “rapidly make informed decisions,” “agile acquisition,” “Rapid fielding” 9, “speed the service’s modernization strategy” 10, and “rapidly providing warfighting capabilities” 11 underscores the central theme: velocity and adaptability are paramount. Traditional, document-intensive engineering and acquisition processes are increasingly recognized as too slow and cumbersome for the modern dynamic threat landscape. Digital Engineering offers a structured pathway to accelerate these processes and enhance responsiveness.
Consequently, the overall success of the broader DoD Digital Engineering Strategy is intrinsically linked to its effective and comprehensive implementation within the T&E domain. The acquisition lifecycle is a continuous process, and DE aims to streamline this entire continuum.1 T&E serves as a critical validation and verification stage within this lifecycle. If T&E processes are not digitally transformed in parallel with design and development efforts, they risk becoming a significant bottleneck, thereby negating the speed and agility gains achieved in earlier phases. The focused attention on DE within DOT&E’s strategic planning 7 clearly acknowledges this critical interdependency and highlights the commitment to ensuring that T&E evolves in lockstep with the rest of the digital enterprise.
C. Foundational Policies: DoD Digital Engineering Strategy and DoDI 5000.97 for T&E
The DoD’s strategic direction for Digital Engineering is anchored by several key documents, with the 2018 DoD Digital Engineering Strategy and the more recent DoD Instruction (DoDI) 5000.97 “Digital Engineering” serving as primary pillars. These policies provide the framework and impetus for transforming T&E practices.
The DoD Digital Engineering Strategy, released in June 2018, laid the groundwork by outlining five strategic goals:
- Formalize the development, integration, and use of models to inform enterprise and program decision-making.
- Provide an enduring, authoritative source of truth (ASoT) for system data and models.
- Incorporate technological innovation to improve engineering practices.
- Establish a supporting infrastructure and collaborative environments.
- Transform the culture and workforce to adopt and support digital engineering across the lifecycle.9 This strategy articulated the “what” of digital engineering, leaving the specific implementation details—the “how”—to the various DoD components and enterprises.9
DoDI 5000.97 “Digital Engineering,” issued in December 2023, represents a significant evolution from strategy to mandate. This instruction establishes formal policy, assigns specific responsibilities, and provides procedures for implementing DE across the DoD.3 A key tenet of DoDI 5000.97 is the requirement for new programs to incorporate digital engineering unless granted an exemption, while existing programs are strongly encouraged to transition towards DE to the maximum extent practical, beneficial, and affordable.5 It fundamentally shifts the primary means of communicating system information from traditional documents to digital models and their underlying data.5
Critically for the T&E community, DoDI 5000.97 specifies that the Director, Operational Test and Evaluation (DOT&E) will utilize digital engineering methods to achieve test objectives for operational assessment and Live Fire Test and Evaluation (LFT&E).12 The instruction also places explicit responsibilities on Program Managers (PMs) to consider the application of DE, ensure that required digital models, artifacts, and data sets are included as contract deliverables, and leverage existing DoD or Service Component-level digital engineering resources before making new investments.5
DoDI 5000.97 introduces a comprehensive framework for digital engineering, which includes several interconnected components:
- Digital Engineering Ecosystem: The overarching infrastructure and architecture.
- Digital Models: Computer-based representations essential for understanding complex systems.
- Digital Twins: Virtual representations of physical systems, updated with real-world data.
- Digital Thread: Connectivity for authoritative data and models across the lifecycle.
- Digital Artifacts: Digital products and views generated from the ecosystem.5
The progression from the 2018 DE Strategy to DoDI 5000.97 signifies a maturing commitment by the DoD. A “Strategy” 9 outlines broad intent and goals, whereas an “Instruction” (DoDI) like 5000.97 5 “establishes policy, assigns responsibilities, and provides procedures.” This transition from the conceptual “what” to a more defined, albeit still needing enterprise-specific tailoring, “how” indicates a higher degree of institutionalization and expectation for DE adoption. The explicit inclusion of DOT&E’s role in utilizing DE methods 12 directly embeds these requirements within the T&E domain.
The components of the DE framework outlined in DoDI 5000.97 are not standalone elements but are deeply interwoven. The digital engineering ecosystem provides the environment for creating and managing digital models. These models can evolve into more sophisticated digital twins, which are dynamic, data-fed representations of specific physical assets.5 The digital thread serves as the connective tissue, linking these models, their underlying data, and various lifecycle activities.5 Digital artifacts, such as test plans or performance reports, are then dynamically generated from these interconnected models and data.5 This interconnectedness is vital for a holistic T&E approach, as T&E activities will leverage all these components: testing models directly, using digital twins for complex scenario evaluations, relying on the digital thread for ensuring traceability of requirements to test results, and evaluating digital artifacts produced throughout the system’s lifecycle.
Table 1: Foundational DoD Digital Engineering Directives and Strategies for T&E
Document Title | Issuing Authority/Date | Primary Objective(s) | Specific Implications/Mandates for T&E |
DoD Digital Engineering Strategy | OUSD(R&E) / June 2018 | Formalize model use, provide ASoT, incorporate innovation, establish infrastructure, transform culture/workforce for DE. 9 | Promote use of digital representations for T&E planning and execution; establish ASoT for test data and models; encourage innovation in T&E methods. |
DoDI 5000.97 “Digital Engineering” | DoD / December 2023 | Establish policy, assign responsibilities, and provide procedures for implementing DE; shift from documents to digital models/data. 3 | Mandates DE for new programs; DOT&E to use DE methods for OT & LFT&E; PMs to include digital deliverables for T&E; leverage digital ecosystem, models, twins, thread, artifacts for T&E. 5 |
DOT&E Strategy Update 2022 (and I-Plan 2023) | DOT&E / 2022 (I-Plan April 2023) | Transform T&E infrastructure, tools, processes, workforce to counter advanced threats and accelerate delivery of working weapons. 7 | Develop digital/model-based TEMPs & IDSKs; integrate T&E with MBSE (“Shift Left”); increase use of credible digital twins in T&E; evaluate AI systems’ operational/ethical performance; improve T&E data management. 7 |
U.S. Army Digital Engineering Policy | U.S. Army / May 2024 10 | Rapid adoption of modern DE practices to speed modernization, identify cost drivers early, and deliver capabilities faster. 10 | Implies Army T&E organizations must adopt DE practices, align with Army DE goals, and leverage digital tools/models for T&E activities to support accelerated modernization. |
II. Anatomy of the DoD T&E Digital Engineering Ecosystem
The Digital Engineering Ecosystem (DEE) for Department of Defense (DoD) Test and Evaluation (T&E) is a complex, interconnected system comprising several core elements. Adapting the generic components of a DEE—Stakeholders, Processes, Tools, Infrastructure, and Standards—to the specific context of DoD T&E reveals a tailored architecture designed to support the rigorous demands of verifying and validating defense systems. This section dissects these elements, highlighting their unique characteristics and interdependencies within the DoD T&E landscape.
Table 2: Core Elements of the DoD T&E Digital Engineering Ecosystem
Ecosystem Element | DoD T&E Specific Description | Primary Function in T&E DEE | Key Examples/Components |
Stakeholders | Includes DOT&E, Service T&E Commands (ATEC, AFOTEC, etc.), Program Managers, Chief Developmental Testers, Warfighters, Defense Industrial Base, Intelligence Community, TRMC, academia. 2 | Define T&E requirements, plan & execute tests, provide resources & infrastructure, analyze results, inform decisions, develop & provide DE tools/methods. | T&E WIPTs, OUSD(R&E), PEOs, Combatant Commanders, FFRDCs. |
T&E Processes | Digitally transformed versions of traditional T&E planning, execution, analysis, and reporting; includes integrated testing (CT, DT, OT, LFT&E), MB-TEMP, IDSK, continuous V&V, Agile/DevSecOps. 11 | To plan, execute, analyze, and report T&E activities using digital methods, enabling ‘Shift Left,’ continuous V&V, and data-driven decisions. | Requirements validation, test scenario development using models, virtual testing, live fire testing augmented by digital data, continuous software testing, mission-based risk assessments. |
Tools & Platforms | Suite of software and hardware for M&S, MBSE, data analytics, AI/ML T&E, digital twin creation/management, PLM/QMS, CI/CD pipelines, and collaboration. 3 | To create, manage, analyze, and visualize digital models, simulations, test data, and digital artifacts; automate processes; facilitate collaboration. | JSE, Advana, JATIC, SysML tools (e.g., Rhapsody), PLM systems (e.g., Teamcenter), Python (e.g., CODEX), TENA, JMETC. |
Infrastructure | Secure, connected IT networks, computing resources (HPC, cloud), data storage, cybersecurity measures, and the digital capabilities of the Major Range Test and Facility Base (MRTFB). 5 | To provide the foundational computing, network, storage, and security capabilities necessary to operate DE tools, manage data, and enable collaboration. | DoD enterprise cloud services, on-premise HPC clusters, secure data centers, MRTFB instrumentation and data networks, DevSecOps environments. |
Standards & Governance | Data/model/interoperability standards (e.g., VAULTIS, SysML, ONNX), security protocols (Zero Trust), process frameworks (Agile), and governance for ASoT and DE practices. 6 | To ensure consistency, interoperability, security, and trustworthiness of data, models, and processes across the ecosystem; guide DE implementation. | DoD Data Strategy, DoDI 5000.97, ISO standards, NIST cybersecurity frameworks, ASoT governance charters, data exchange agreements. |
A. Key Stakeholders and Collaborative Networks
The DoD T&E DEE is characterized by a diverse and extensive network of stakeholders, each playing a critical role in its functioning and success. These stakeholders span leadership, policy-making bodies, program execution offices, T&E practitioner organizations, end-users, industry partners, and the intelligence community.
- DoD Leadership and Policy Bodies: At the highest level, the Office of the Under Secretary of Defense for Research and Engineering (OUSD(R&E)) provides overarching guidance for DE strategy.3 The Director, Operational Test and Evaluation (DOT&E) is paramount in setting T&E policy, driving the strategic transformation of T&E capabilities, and ensuring DE methods are utilized for operational assessments and LFT&E.7 Service Acquisition Executives and Service Operational Test Commanders are also key in translating these policies into actionable plans within their respective services.7
- Program-Level Entities: Program Managers (PMs) bear the direct responsibility for implementing DE within their specific acquisition programs, including the digital aspects of T&E.5 They work closely with Program Executive Offices (PEOs), Chief Developmental Testers (CDT), and T&E Working Integrated Product Teams (T&E WIPTs), which serve as crucial collaborative forums for planning and overseeing T&E activities.15
- T&E Practitioner Organizations: The actual execution and specialized support for T&E are provided by Service T&E organizations such as the Army Test and Evaluation Command (ATEC) 19, Air Force Operational Test and Evaluation Center (AFOTEC), the Navy’s Operational Test and Evaluation Force (OPTEVFOR), the Marine Corps Operational Test and Evaluation Activity (MCOTEA), and the Joint Interoperability Test Command (JITC).11 The Test Resource Management Center (TRMC) plays a vital role in managing investments in T&E infrastructure, including digital capabilities, and overseeing initiatives like TENA and JMETC.7
- End Users and Operational Forces: Warfighters provide essential operational context and feedback throughout the T&E process, ensuring that systems are evaluated against realistic mission scenarios.1 Combatant Commanders (COCOMs) offer critical input on mission threads and operational needs, which inform T&E planning and evaluation criteria.7
- Industry and Academia: The Defense Industrial Base (DIB) comprises crucial partners in developing systems and implementing DE practices, often working collaboratively with DoD entities.2 Technology vendors supply many of the specialized tools and platforms used within the DEE.10 Academic institutions and Federally Funded Research and Development Centers (FFRDCs), such as the Systems Engineering Research Center (SERC), MITRE, and The Aerospace Corporation, contribute through research, development of new methodologies, and independent assessments.2
- Intelligence Community (IC): The IC is a close and indispensable partner, providing critical threat intelligence to inform T&E scenarios, contributing to the development of realistic threat models for simulations, and assisting in the protection of sensitive DE artifacts and data within the ecosystem.2
The generic DEE image provided in the user query, which depicts stakeholders like Engineering Teams, Project Management, Clients & End Users, Quality Assurance, and DevOps (Sec) Operations, maps effectively to these specific DoD T&E stakeholders. For example, “Engineering Teams” encompass both government and industry developers and testers, “Project Management” aligns with PMs and PEOs, and “Clients & End Users” directly correspond to Warfighters and COCOMs.
The sheer diversity and distributed nature of these stakeholders underscore a fundamental characteristic of the T&E DEE: its success is critically dependent on achieving unprecedented levels of collaboration and seamless data sharing across numerous organizational and contractual boundaries. This presents a significant cultural and technical hurdle. The effective functioning of a DEE, particularly one supporting T&E, requires breaking down traditional silos and fostering an environment of shared understanding and trust, facilitated by common data standards and interoperable tools. The explicit calls in strategic documents for the T&E community to work together as a “unified enterprise” 7 and the acknowledgment of existing silos as a challenge 11 highlight this imperative.
Furthermore, the advent of DE is actively reshaping the traditional roles and responsibilities of these T&E stakeholders. The “Shift Left” paradigm, for example, which advocates for integrating T&E considerations much earlier in the system development lifecycle 7, means that testers and evaluators must engage with digital models and simulations far sooner than they would with physical prototypes in a traditional process. This requires new skills and a different mindset. Similarly, PMs now have explicit new responsibilities under DoDI 5000.97, such as ensuring that digital models, artifacts, and datasets are included as contract deliverables and that appropriate data rights are secured.5 This represents a new focus area for program management, demanding a deeper understanding of digital engineering principles and practices. These evolving roles necessitate significant investment in workforce retraining, the development of new competencies, and a deliberate effort to adapt organizational cultures to the new digital ways of working.
B. Digitally Transformed T&E Processes
Digital Engineering is not merely about applying new tools to old processes; it is about fundamentally transforming the T&E processes themselves. This transformation aims to make T&E more integrated, agile, data-driven, and continuous throughout the system lifecycle.
- Lifecycle Integration: DE principles advocate for supporting activities from the initial concept phase all the way through system disposal.1 This holistic view means that T&E processes, including Contractor Testing (CT), Developmental Testing (DT), Operational Testing (OT), and Live Fire Test and Evaluation (LFT&E), are increasingly being integrated and informed by digital engineering practices and data streams.11 The goal is to create a continuum of evaluation rather than a series of disconnected test events.
- Model-Based Test and Evaluation Master Plan (MB-TEMP) and Integrated Decision Support Key (IDSK): A cornerstone of this process transformation is the shift away from static, document-centric Test and Evaluation Master Plans (TEMPs) towards dynamic, Model-Based TEMPs (MB-TEMPs).11 These MB-TEMPs are living digital artifacts, often directly linked to system models and requirements databases. A key component of the MB-TEMP is the Integrated Decision Support Key (IDSK), a structured framework that explicitly links programmatic decisions (e.g., milestone approvals, production readiness) to specific evaluation criteria, measures of performance, and the underlying test data required to inform those decisions.15
- “Shift Left” and Integrated Testing: A significant process change enabled by DE is the concept of “Shift Left,” which involves integrating T&E activities much earlier in the development lifecycle.7 By leveraging digital models and simulations, T&E can begin during the design phase, allowing for early identification of issues, validation of requirements, and assessment of design alternatives. This includes exposing early system prototypes or concepts to operational contexts within sophisticated digital environments, long before physical hardware is available.11
- Continuous Verification and Validation (V&V): DE facilitates a move towards more frequent and thorough testing throughout the development process, rather than relying on a few major test events.3 For software-intensive systems and particularly for AI-enabled systems, continuous evaluation of operational performance, safety, and ethical considerations is heavily emphasized.7 This continuous approach allows for iterative refinement and rapid feedback loops.
- Data-Driven Decision Making: A central theme in the transformation of T&E processes is the emphasis on using robust test data and validated models to inform critical acquisition and fielding decisions.9 The entire DEE is geared towards generating, managing, and analyzing data to provide decision-makers with timely and credible insights.
- Agile and DevSecOps in T&E: For software-reliant systems, DE in T&E aligns closely with Agile and DevSecOps methodologies. This involves automating testing processes, enabling rapid data reduction and analysis, and supporting continuous software integration and distribution throughout the system’s lifecycle.5
When comparing these transformed T&E processes to the generic process flow depicted in the provided image (Requirements Gathering, Design Phase, Development & Implementation, Simulation & Testing, Deployment, Maintenance & Support), it’s clear that DE has a pervasive impact. While “Simulation & Testing” is the most direct parallel, DE influences T&E considerations in all phases. For instance, requirements must be defined in a testable manner (often captured in models), the design phase incorporates early M&S for T&E purposes, and data from deployed systems feeds back into the DEE for continuous monitoring and support of T&E for future upgrades.
This evolution from traditional, often sequential and document-heavy T&E processes to dynamic, model-based, and data-centric approaches represents a genuine paradigm shift. The traditional TEMP, as described in sources like 24 and 22, is typically a static document outlining a future test strategy. In contrast, an MB-TEMP is conceived as a living digital artifact, directly connected to system models, requirements databases, and evolving test data.11 This is not merely a change in format (e.g., from paper to PDF) but a fundamental change in how T&E is planned, managed, and integrated with the broader engineering effort. Similarly, the “Shift Left” philosophy 7 alters when and how T&E professionals engage in the acquisition process, moving them from being primarily validators of physical prototypes to active participants in early design validation using digital tools.
Underpinning these transformed T&E processes is the critical role of data. The entire digital T&E paradigm is predicated on the availability, accessibility, quality, and effective flow of data. The IDSK, for example, explicitly functions by linking decisions directly to supporting data.15 The ability to make data-driven decisions 9 relies on the integrity and trustworthiness of that data. The “predict, live test, refine” feedback loop envisioned for M&S in T&E 11 is entirely dependent on robust data exchange between simulation environments and live test data sources. This profound reliance on data elevates the importance of comprehensive data management strategies and the establishment of an Authoritative Source of Truth (ASoT) as the veritable backbone of the T&E DEE.
C. Essential Tools and Platforms
The execution of digitally transformed T&E processes relies on a diverse and evolving suite of specialized tools and platforms. These digital capabilities are essential for creating, managing, and analyzing the models, simulations, and data that form the core of the T&E DEE.
- Modeling and Simulation (M&S) Tools: M&S is a foundational element for DE in T&E.3 This category encompasses a wide range of tools used to create system performance models, physics-based simulations of components or environments, and operational environment models that represent combat scenarios. These tools are employed for early analytical studies, virtual testing of system capabilities, augmenting live test events by providing context or stimuli, and exploring scenarios that are too costly, dangerous, or complex for purely physical testing.11
- A prominent example is the Joint Simulation Environment (JSE), an advanced, government-owned digital battlespace specifically designed for the integrated test of multi-domain systems. Unlike many legacy simulation environments adapted from training applications, JSE is engineered from the ground up to support rigorous T&E activities, leveraging authoritative threat models from Intelligence Community partners.25
- Model-Based Systems Engineering (MBSE) Tools: These are software applications used for creating, managing, analyzing, and visualizing system models, often based on standardized languages like the Systems Modeling Language (SysML). Tools such as Rhapsody (mentioned in the context of Raytheon’s MBSE efforts 16) enable engineers and testers to define system architectures, requirements, behaviors, and interfaces in a structured, model-based format.
- Data Analytics and AI/ML Platforms: Given the vast amounts of data generated by digital T&E activities, tools for processing, analyzing, and visualizing this data are crucial. This includes platforms for statistical analysis, data mining, and increasingly, Artificial Intelligence (AI) and Machine Learning (ML) applications.
- The Advana Platform serves as the DoD’s enterprise data and analytics environment, providing access to data from numerous DoD business systems and a suite of analytical tools.27
- The Joint AI Test Infrastructure Capability (JATIC) is specifically focused on developing cutting-edge software solutions to advance AI T&E and AI Assurance across the DoD.27
- Specialized tools like CODEX (Coverage of Data Explorer), a Python-based toolkit, are being developed to implement metrics and algorithms for assessing data coverage in the context of AI/ML T&E.11
- Digital Twin Platforms: These are software environments designed to create, manage, and operate digital twins. They integrate data from physical assets with their digital models, enabling real-time monitoring, performance prediction, and “what-if” scenario analysis.5
- Product Lifecycle Management (PLM) and Quality Management Systems (QMS): PLM systems are used to manage all information about a product throughout its lifecycle, from concept to disposal. QMS tools help ensure adherence to quality standards. These systems are increasingly being integrated with DE tools and processes to provide a comprehensive digital backbone for product development and sustainment.28 Teamcenter is an example of a PLM system used in the defense industry.13
- Continuous Integration/Continuous Delivery (CI/CD) Tools: For software-intensive systems, CI/CD pipelines are essential for automating the build, test, and deployment processes. These tools are fundamental to implementing DevSecOps practices within the T&E domain, enabling rapid and continuous testing of software updates.5
- Collaboration Platforms: Given the distributed nature of T&E stakeholders, platforms that enable secure sharing of models, data, documents, and other digital artifacts are critical for effective teamwork.9
- Test Resource Management Center (TRMC) Initiatives: The TRMC sponsors and oversees several key enabling architectures and capabilities, including the Test and Training Enabling Architecture (TENA), which provides a common framework for range interoperability, and the Joint Mission Environment Test Capability (JMETC), which facilitates distributed testing across multiple locations.20
The generic DEE image’s depiction of tools such as Development Tools, Modeling & Simulation, Data Analytics, CI/CD Tools, and Monitoring Tools aligns well with these specific DoD T&E capabilities.
A critical factor for the success of the T&E DEE is the interoperability of this diverse tool suite. The ecosystem relies on data flowing seamlessly between MBSE tools, M&S environments, data analytics platforms, and other specialized applications. However, a lack of interoperability is a frequently cited challenge.1 If these tools cannot exchange data effectively, it leads to manual data re-entry, the need for complex and costly custom interfaces, significant inefficiencies, and ultimately undermines the core concept of an integrated digital thread. Recognizing this, the DoD’s digital engineering strategy emphasizes a focus on common standards, data formats, and interfaces between tools, rather than mandating specific tools.9 This approach allows for flexibility in tool selection while promoting the necessary data exchange capabilities.
While many DE tools have general applicability, the unique and demanding requirements of DoD T&E are driving the emergence of specialized tools and platforms. JSE, for example, is explicitly “designed from the ground up for test, not only training” 25, highlighting the distinct needs of the T&E community for rigor, fidelity, and specific analytical capabilities. Similarly, JATIC’s focus on “AI T&E and AI Assurance” 27 addresses the novel challenges posed by testing AI-enabled systems, such as evaluating their robustness, resiliency, explainability, and ethical implications. This trend towards specialized T&E tooling indicates a growing recognition that off-the-shelf commercial tools may not always suffice for the complex, high-consequence systems evaluated by the DoD, necessitating targeted investments in T&E-specific digital capabilities.
D. Supporting Infrastructure
The diverse tools and complex processes of the T&E DEE are reliant upon a robust and secure supporting infrastructure. This infrastructure encompasses the foundational hardware, software, networks, and physical facilities necessary to enable digital engineering practices across the T&E lifecycle.
- Digital Engineering IT Infrastructures: This is a broad category that includes the collection of hardware, software, networks, and related equipment, often spanning multiple geographical locations and organizations. A key requirement is that this infrastructure must satisfy stringent security protocols to protect sensitive T&E data and intellectual property.9
- Secure Connected Information Networks: Reliable, available, and secure information networks are fundamental for the flow of digital information within the T&E DEE. These networks must support operations at all necessary classification levels and facilitate seamless communication and data exchange among distributed stakeholders.9
- Computing Resources: The T&E DEE demands significant computational power. This includes scalable hardware solutions, potentially incorporating High-Performance Computing (HPC) for complex simulations and data analysis 29, and a range of software solutions. The DoD is increasingly considering the use of commercial cloud platforms and service solutions where appropriate to provide flexible scalability and potentially rapid deployment of capabilities.9
- Major Range Test and Facility Base (MRTFB): The physical test ranges and facilities that constitute the MRTFB are critical components of the T&E infrastructure. Importantly, DoDI 5000.97 directs Program Managers to leverage the digital engineering infrastructure capabilities of the MRTFB.5 The TRMC plays a significant role in overseeing investments in and the modernization of T&E infrastructure, including the digital capabilities of the ranges.20 The DOT&E strategy further emphasizes standardizing the digital representation of the joint, multi-domain operating environment, which directly impacts the digital capabilities and connectivity of the MRTFB.7
- Data Storage and Management Infrastructure: Secure and scalable repositories are required for storing the vast quantities of models, simulation data, test results, and other digital artifacts generated within the DEE.7 This includes capabilities for data curation, configuration management, and long-term archival.
- Cybersecurity Infrastructure: Given the sensitivity of the information handled within the T&E DEE, a robust cybersecurity infrastructure is paramount. This is essential to protect models, data, and the DEE itself from both internal and external threats, ensuring the integrity, availability, and confidentiality of T&E information.9
The generic DEE image’s infrastructure components—Cloud Platforms, On-Premise Service, IoT Systems—provide a relevant, albeit high-level, starting point for understanding the DoD T&E context.
The MRTFB is evolving from being merely a collection of physical test locations to a critical digital infrastructure node within the broader T&E DEE. The directive for PMs to “Leverage Major Range Test and Facility Base digital engineering infrastructure capabilities” 5 and DOT&E’s strategic goal to create a “common, digitized, and transparent picture of existing and future range capabilities” 7 clearly indicate this shift. This means that range instrumentation, data collection networks, local simulation environments, and data processing capabilities at the MRTFB sites must be modernized and integrated with the enterprise-level DEE. Ranges are thus becoming digitally connected hubs that support both live and virtual testing, feeding critical data into the Authoritative Source of Truth and enabling more comprehensive and integrated evaluations.
Given the diverse requirements of the T&E DEE, particularly concerning security classifications, the need to interface with legacy systems, and the demand for specialized high-performance computing for certain M&S tasks, the supporting infrastructure will inevitably be a hybrid model. This will likely involve a carefully orchestrated mix of on-premise government-owned systems, secure government cloud environments 28, and potentially commercial cloud services for less sensitive applications or specific capabilities.9 The need to provide “enterprise services at all classification levels” 9 reinforces the necessity of this multifaceted and adaptable infrastructure strategy. Highly sensitive T&E data or specialized, resource-intensive range systems may remain on-premise or within dedicated government enclaves, while other analytical tools, collaboration platforms, or development environments could leverage various cloud solutions to achieve the desired balance of capability, security, and cost-effectiveness.
E. Governing Standards and Frameworks
For the T&E DEE to function effectively as an integrated and trustworthy system, it must be governed by a comprehensive set of standards and frameworks. These standards ensure consistency, interoperability, security, and quality across the diverse tools, processes, and data that comprise the ecosystem.
- Data Standards: These are crucial for enabling interoperability and ensuring that data is VAULTIS (Visible, Accessible, Understandable, Linked, Trustworthy, Interoperable, Secure).6 Adherence to the goals of the DoD Data Strategy is paramount in this regard.6 Standardized data formats, metadata schemas, and data exchange protocols are essential for data to flow seamlessly and be usable across different platforms and by various stakeholders.
- Model Standards: Formalisms for model development, including defined syntax, semantics, and lexicons, are necessary to ensure that models are unambiguous, reusable, and can be reliably integrated.9 For example, SysML is a common standard for systems modeling, and ONNX (Open Neural Network Exchange) is mentioned as a standard for representing AI models in the context of T&E.11
- Interoperability Standards: A primary focus of the DoD’s DE strategy is on establishing standards, data formats, and interfaces between tools, rather than mandating specific tools.9 This approach promotes flexibility while ensuring that different tools can effectively exchange information and work together within the ecosystem.
- Security Standards and Protocols: Protecting intellectual property, ensuring cybersecurity, and maintaining appropriate security classification for models and data are critical.9 This includes compliance with overarching DoD security directives, such as DoDD 5205.02E concerning Operational Security 5, and the incorporation of modern security paradigms like Zero Trust principles into DE solutions and infrastructure.10
- Process Frameworks: The adoption of standardized process frameworks, such as Agile methodologies and DevSecOps practices, helps to ensure consistency and efficiency in how DE and digital T&E are implemented, particularly for software development and testing.5
- Governance Frameworks: Formal governance structures and processes are essential, particularly for managing the Authoritative Source of Truth (ASoT).9 This includes defining roles, responsibilities, policies, and procedures to ensure that models and data are formally managed, trusted, and maintained throughout their lifecycle.
- ISO Standards: While mentioned generally in the provided DEE image, specific ISO (International Organization for Standardization) standards relevant to systems engineering (e.g., ISO/IEC/IEEE 15288), software engineering, data quality (e.g., ISO 8000 series), and information security management (e.g., ISO 27000 series) would be applicable and beneficial within the T&E DEE.
The generic image’s categories of ISO Standards, Agile/Scrum Methodologies, and Security Standards are well-represented and expanded upon in the DoD T&E context.
The establishment and consistent enforcement of robust standards, especially those pertaining to data and model interoperability, are fundamental to the T&E DEE’s ability to achieve the necessary scale, trustworthiness, and operational efficiency. These standards form the bedrock of a federated yet coherent ecosystem, allowing diverse components to interact reliably. The DoD strategy’s explicit focus on “standards, data, formats, and interfaces between tools rather than being constrained to particular tools” 9 underscores this principle. Tools and technologies will inevitably evolve, but enduring standards ensure the long-term usability of data, the ability to integrate new capabilities, and the consistent application of best practices. The VAULTIS principles 6, for example, effectively serve as a high-level standard for data quality and accessibility, which is indispensable for credible T&E and informed decision-making.
However, there exists an inherent tension between the need for standardization and the imperative to incorporate “technological innovation to improve the engineering practice,” which is Goal 3 of the DoD Digital Engineering Strategy.9 While standards are crucial for stability and interoperability, overly rigid or slow-to-evolve standards could inadvertently stifle the adoption of cutting-edge DE and T&E tools, methods, and technologies. The DE landscape is characterized by rapid evolution, particularly in areas like AI/ML.11 If standards development and adoption processes cannot keep pace with this innovation, or if standards become excessively prescriptive, they risk becoming barriers rather than enablers. Therefore, the T&E DEE requires a balanced approach, potentially involving adaptable “living standards,” modular architectures, and frameworks that can accommodate and integrate innovation while maintaining core principles of interoperability and trustworthiness.
III. Core Enablers of the T&E Digital Engineering Ecosystem
The functionality and transformative potential of the DoD Test & Evaluation (T&E) Digital Engineering Ecosystem (DEE) are realized through several core technological and methodological enablers. These elements work synergistically to create an environment where T&E can be conducted more efficiently, effectively, and with greater insight. Key among these are the Authoritative Source of Truth (ASoT), Digital Models, Digital Twins, the Digital Thread, Model-Based Systems Engineering (MBSE), and the expanding role of Artificial Intelligence and Machine Learning (AI/ML).
A. The Authoritative Source of Truth (ASoT) as the T&E Data Backbone
The Authoritative Source of Truth (ASoT) is a foundational concept within the DoD’s Digital Engineering Strategy and a critical enabler for the T&E DEE. It is defined as the designated, configuration-controlled reference point for all relevant models and data pertaining to a system throughout its entire lifecycle.30 The ASoT’s primary role is to provide unambiguous traceability as the system evolves, meticulously capturing historical knowledge, and connecting authoritative versions of diverse models and datasets.9 This ensures that all authorized stakeholders have access to current, consistent, and validated information, forming the bedrock for reliable analysis and decision-making.9 Goal 2 of the DoD Digital Engineering Strategy explicitly mandates efforts to “Provide an enduring, authoritative source of truth” 9, and DoDI 5000.97 further directs programs to establish an ASoT for their data and models.5
Within the T&E domain, the ASoT serves as the central data backbone, equipping programs with the enterprise-wide knowledge necessary for comprehensive T&E planning, efficient execution, robust analysis, and informed sustainment strategies.9 It is the trusted source from which digital artifacts related to T&E are generated, technical reviews are supported, and critical decisions regarding system performance, suitability, and readiness are informed.9 Data from all T&E activities—including contractor tests, developmental tests, operational tests, live-fire events, and modeling and simulation efforts—are intended to feed into, be managed by, and remain traceable within this ASoT.
The establishment and maintenance of an ASoT are not merely technical endeavors; they require robust governance. This includes clearly defined policies, stringent procedures, carefully managed access controls, and diligent data management practices to ensure the ongoing integrity, quality, and trustworthiness of the information it contains.9 It is important to recognize that the ASoT does not represent an absolute, immutable truth, but rather a proclaimed and validated source of information deemed authoritative by the governing body.18 Typically, there is a one-to-many relationship, where a single ASoT framework can manage and provide access to numerous digital artifacts.18
The ASoT’s role extends beyond being a mere data repository; it is the fundamental enabler of truly integrated T&E. Current T&E practices often suffer from data existing in isolated silos, frequently residing in “disparate retrograde spreadsheets” 11, which hinders comprehensive analysis and knowledge sharing. An ASoT, by its very definition, provides a “common set of digital models and data” 9 and actively “connects authoritative versions of models and data”.30 This inherent connectivity is essential for realizing a “whole body of evidence” approach to T&E, where insights from all test phases and data sources are combined.11 It also facilitates the crucial capability of carrying knowledge forward across different test phases and throughout the system’s lifecycle 11, ensuring that lessons learned and performance data from early M&S or DT activities directly inform subsequent OT and fielding decisions.
While the technical challenges of implementing an ASoT are considerable, the effectiveness of its governance framework is arguably even more critical and complex. The success of the ASoT hinges on establishing and enforcing clear rules for data ownership, stewardship responsibilities, access permissions, data quality standards, and configuration management.918 extensively details the governance challenges, including identifying the entities with authority over data, managing Systems of Record (SoRs), defining the roles of originators and owners, preventing the proliferation of “rogue sources” of data, and ensuring appropriate stakeholder access. Similarly, 9 emphasizes the role of governance in maintaining the integrity and quality of the ASoT. If stakeholders lose trust in the data contained within the ASoT due to perceived or actual failures in governance, they will inevitably revert to relying on their own local or private data sources. This would lead to the fragmentation of the T&E data landscape, thereby undermining the core purpose of the ASoT and diminishing the effectiveness of the entire DEE.
B. Leveraging Digital Models, Digital Twins, and the Digital Thread for T&E
Digital Models, Digital Twins, and the Digital Thread are interconnected concepts that form the core technical fabric of the T&E DEE, enabling advanced methods of system evaluation and lifecycle management.
- Digital Models: These are computer-based representations of an object, phenomenon, process, or system, which may include its form, attributes, functions, and behavior, often depicted visually or as mathematical or logical expressions.5 Within the DoD, digital models are considered “essential to understanding complex systems and system interdependence and to communicate among team members and stakeholders”.6 A key requirement is that these models and their underlying data must be traceable across the entire system lifecycle, “from operational capabilities through requirements, design contracts, production, test, training, and sustainment”.6 They are extensively used for simulation, virtual testing, and to support manufacturing processes.17
- Digital Twins: A digital twin is a more advanced and dynamic form of a digital model. It is a virtual representation of a specific real-world physical asset, system, or process that is kept synchronized with its physical counterpart through the flow of data.5 This connection allows the digital twin to mirror and predict the activities, performance, and health of its physical twin.5 In the DoD context, digital twins are being applied to improve design, enhance testing capabilities, optimize maintenance schedules, and manage system performance throughout the lifecycle (e.g., optimizing F-35 radar settings for specific missions).17 For T&E, digital twins can also represent elements of the test environment itself, such as a digital twin of a test range.14
- Digital Thread: The digital thread is the connective infrastructure that links authoritative data and digital models (including digital twins) across organizations and throughout a system’s lifecycle, providing actionable information to decision-makers.5 It enables robust traceability, allowing engineers and testers to understand the impact of changes and to follow the lineage of requirements, design decisions, and test results.17 The digital thread effectively forms the information infrastructure that underpins and provides access to the Authoritative Source of Truth.16
In the T&E domain, these enablers are transforming practices. Models and simulations are becoming primary means of information exchange, augmenting or sometimes replacing traditional document-based approaches.31 Digital twins offer the capability to conduct testing in environments where physical testing might be constrained due to cost, safety, security, or ethical considerations.14 The digital thread ensures that test plans are linked to system configurations, requirements are traceable to test results, and that a continuous record of system performance and evaluation is maintained.
Digital Twins, in particular, offer the potential to revolutionize the scope and fidelity of T&E. Unlike generic digital models which often represent a system at a “type or class level,” a digital twin is tied to a “specific individual unit” of that type (e.g., a specific aircraft tail number or ship hull number).14 This specificity, combined with the automated, often bi-directional, flow of data from the physical asset 14, allows T&E to move beyond evaluating general system performance characteristics to understanding the nuanced behavior and condition of individual operational assets. This capability can support highly precise performance assessments, generate data for predictive maintenance strategies that are directly relevant to operational suitability evaluations, and enable the testing of complex “what-if” scenarios on a virtual representation of an actual fielded system—scenarios that might be impossible or unacceptably risky to conduct in live settings. The F-35 example, where a digital twin is used to determine optimal radar settings for different missions and regions 17, illustrates this potential for tailored, data-driven performance optimization and evaluation.
The Digital Thread, meanwhile, is what makes the concept of “continuous V&V” and true lifecycle T&E a practical reality. Without the persistent, traceable connections provided by the digital thread, each T&E phase or event risks becoming an isolated activity, with valuable knowledge lost or difficult to transfer to subsequent phases or to operational support. The digital thread “connects authoritative data and digital models…throughout a system’s life cycle” 5, creating an unbroken chain of information. This allows, for instance, an operational deficiency discovered in the field to be traced back through the thread to specific design elements, component specifications, or earlier test results, thereby facilitating rapid root cause analysis and the efficient verification of corrective actions. The requirement that models and their data be traceable “from operational capabilities through requirements, design contracts, production, test, training, and sustainment” 6 explicitly mandates this level of lifecycle continuity, which the digital thread is designed to provide.
C. Model-Based Systems Engineering (MBSE) as a Catalyst for T&E Transformation
Model-Based Systems Engineering (MBSE) is a formalized methodology involving the application of modeling to support the full spectrum of systems engineering activities. This includes requirements definition and analysis, design synthesis, system analysis, and verification and validation, starting from the conceptual design phase and continuing throughout development and into the later lifecycle phases.16 MBSE is not merely the use of models, but a disciplined approach that leverages models as the primary artifacts for communication, analysis, and documentation.
Within the broader Digital Engineering strategy of the DoD, MBSE is recognized as a key approach and a foundational element.17 It serves to transform traditional, often cumbersome, document-based engineering stovepipes into more agile, product-centric, and integrated digital enterprises.31
For the T&E community, MBSE offers significant benefits. It has the potential to improve overall system quality, enhance the consistency of system representations, and provide robust digital traceability from requirements through to test verification.31 This, in turn, can lead to increased personnel productivity, earlier identification of risks and system defects, reductions in development costs and schedules, improvements in system performance, and significantly enhanced communication and collaboration among diverse stakeholder groups.31 MBSE directly supports the implementation of frameworks like the Developmental Evaluation Framework (DEF), providing a structured, model-based way to articulate the T&E strategy and link it to program decisions.16 Furthermore, MBSE enables the systematic decomposition of complex test events into manageable test cases and supports automated impact analysis of proposed system changes on the test program.16 The models created through MBSE practices are primary contributors to the Digital Thread and populate the Authoritative Source of Truth, forming the core of the system’s digital representation.16
MBSE, particularly when utilizing standardized modeling languages such as SysML, effectively provides a common descriptive framework—a shared “language”—for the T&E DEE. This common language allows diverse T&E stakeholders, including systems engineers, software developers, test planners, evaluators, program managers, and operational users, to communicate clearly and unambiguously about the system under test, its requirements, architecture, interfaces, and expected behaviors. Traditional T&E often relies heavily on natural language descriptions in documents, which can be prone to ambiguity, misinterpretation, and inconsistency. In contrast, MBSE employs formal models with defined syntax and semantics.9 These models become the precise and authoritative reference for T&E planning (e.g., deriving specific test cases directly from model elements or behavioral diagrams) and for T&E analysis (e.g., comparing observed test results against model-predicted behaviors). The assertion that MBSE uses models as the “primary means of information exchange” 31 underscores this shift towards a more rigorous and less ambiguous form of technical communication.
Moreover, the integration of T&E considerations into early-phase system models via MBSE practices facilitates the concept of designing systems to be “testable by design.” This means that testability requirements, necessary observation points for data collection, built-in-test (BIT) capabilities, and specific evaluation criteria are considered and incorporated into the system architecture from the very beginning of the development lifecycle, rather than being addressed as an afterthought or retrofitted late in the process. MBSE supports engineering activities “beginning in the conceptual design phase”.31 If T&E stakeholders actively participate in this early MBSE modeling process, aligning with the “Shift Left” philosophy, they can ensure that the system model itself includes elements that directly facilitate subsequent testing and evaluation. For example, they can advocate for the definition of specific data interfaces for test instrumentation or the inclusion of verifiable performance parameters within the model, which then directly drive the physical design and software development. The Developmental Evaluation Framework (DEF), as described in 16, explicitly links evaluation criteria to early program decisions and the models that support them, reinforcing this proactive approach to testability.
D. The Expanding Role of Artificial Intelligence and Machine Learning (AI/ML) in T&E
Artificial Intelligence (AI) and Machine Learning (ML) are rapidly emerging as transformative technologies with a dual impact on the DoD T&E DEE: they present new and complex systems that require robust T&E, and they offer powerful new tools to enhance the T&E process itself.
- T&E of AI-Enabled Systems: The evaluation of AI-enabled systems is a major focus area for DOT&E and the broader T&E community. These systems often exhibit complex, adaptive, and sometimes non-deterministic behaviors, posing unique challenges for traditional T&E methodologies. There is a strong emphasis on the need for continuous evaluation of their operational performance, safety, reliability, and ethical implications, even after deployment.7 Key challenges in testing AI systems include their operational scale, the accessibility of internal states for verification, and the need for efficient and adaptive test processes.11
- AI/ML Tools for Enhancing T&E Processes: Significant research and development are underway to create AI/ML-powered tools and techniques to improve T&E. This includes work on hierarchical scoring methods for evaluating classification and object detection algorithms, Systematic Inclusion/Exclusion (SIE) techniques for identifying critical features of a model’s operating envelope, the CODEX tool for assessing data coverage in AI/ML testing, Systems Theoretic Process Analysis (STPA) for AI ethics assessment, and the use of standards like ONNX and SysML for model-based testing of AI systems.11 The Joint AI Test Infrastructure Capability (JATIC) is specifically tasked with developing and providing software solutions to advance AI T&E and AI Assurance across the DoD.27
- AI/ML in T&E Data Analysis: AI and ML algorithms can be employed to analyze the vast and complex datasets generated during T&E. These techniques can help identify subtle patterns, anomalies, and correlations in performance data, support advanced performance inference (e.g., using Bayesian methods), and automate aspects of data reduction and reporting.11
- AI in Modeling and Simulation (M&S): AI can enhance the realism and complexity of M&S environments used for T&E. This can involve using AI to create more intelligent and adaptive adversary behaviors, to model complex environmental factors, or to generate synthetic data for training and testing AI systems themselves.
AI presents both a significant challenge and a substantial opportunity for the T&E DEE. On one hand, T&E processes and methodologies must evolve rapidly to effectively test the increasingly sophisticated AI-enabled systems being developed by the DoD (AI as the subject of T&E). This requires new approaches to V&V, new types of test environments, and new metrics for assessing performance and trustworthiness.7 On the other hand, AI and ML techniques are themselves becoming powerful tools that can be leveraged to enhance the efficiency, depth, and insightfulness of the T&E process itself (AI as a tool for T&E). The development of methods like Bayesian inference for performance characterization and tools like CODEX for data coverage analysis 11 are examples of AI being used to improve how T&E is conducted.
The unique and often formidable challenges associated with testing AI systems—such as dealing with non-determinism, ensuring robustness across vast input spaces, validating data dependencies, and addressing ethical considerations—are actively pushing the boundaries of traditional DE and T&E methodologies. This is compelling innovation in areas such as adaptive experimental design (where the test plan evolves based on observed results), advanced uncertainty quantification techniques, and the development of highly specialized digital tools and virtual environments tailored for AI T&E. For instance, the identification of “Core challenges to adequately test CogEW (Cognitive Electronic Warfare): 1) scale, 2) accessibility, and 3) Efficient test processes and execution,” and the subsequent recommendation to use “adaptative sequential experimental design (ASED)” 11, demonstrates how the inherent nature of AI is forcing the T&E community to move beyond conventional, pre-scripted test plans towards more dynamic, intelligent, and data-driven testing approaches, all managed and executed within a digital framework.
Table 3: Key Digital Enablers and Their Impact on DoD T&E
Digital Enabler | Brief Description in T&E Context | Transformative Impact on T&E Capabilities | Key DoD Policies/Strategies Referencing It |
Authoritative Source of Truth (ASoT) | Central, governed reference for all T&E-related models, data, and artifacts, ensuring traceability and consistency. 9 | Ensures trusted, traceable, and integrated test data across all T&E phases (CT, DT, OT, M&S, LFT&E), supporting holistic evaluation, data-driven decisions, and lifecycle knowledge retention. | DoD DE Strategy (Goal 2) 9; DoDI 5000.97.5 |
Digital Models | Computer-based representations of systems, environments, and processes used for simulation, analysis, and V&V. 5 | Enable early T&E (“Shift Left”), virtual testing of complex scenarios, requirements validation, performance prediction, and reduced reliance on physical prototypes. Supports MB-TEMP and IDSK. | DoD DE Strategy (Goal 1) 9; DoDI 5000.97 5; DOT&E Strategy.7 |
Digital Twins | Dynamic virtual replicas of specific physical assets or systems, continuously updated with real-world data. 5 | Allows T&E of individual operational units, prediction of specific asset performance, “what-if” analysis on fielded systems, and testing in constrained or hazardous environments. Supports continuous monitoring and evaluation. | DoDI 5000.97 5; DOT&E Strategy.7 |
Digital Thread | Connective data flow linking models, data, and processes across the lifecycle, ensuring traceability and integration. 5 | Provides end-to-end traceability from requirements to test results to operational performance. Enables impact analysis of changes, ensures configuration management of test articles, and supports continuous V&V throughout the lifecycle. | DoDI 5000.97.5 |
Model-Based Systems Engineering (MBSE) | Formalized application of modeling to support systems engineering, including T&E planning and V&V activities. 16 | Provides a common “language” for T&E, enables “testable by design,” improves requirements clarity, supports test case derivation from models, facilitates automated impact analysis, and enhances collaboration among T&E stakeholders. | DoD DE Strategy (implicit in model formalization); DoDI 5000.97 (supports model-centric approach); DOT&E Strategy (supports MB-TEMP and integrated T&E). |
Artificial Intelligence/Machine Learning (AI/ML) | Technologies for analyzing complex data, automating tasks, and enabling adaptive system behaviors. 7 | Presents new T&E challenges for AI-enabled systems (requiring new methods for V&V, ethics). Offers powerful tools for T&E data analysis, automated test generation, intelligent M&S, and adaptive experimental design. Supports T&E of complex, evolving systems. | DOT&E Strategy (Pillar 4 – evaluate AI systems) 7; Various research initiatives (JATIC, SERC projects).11 |
IV. Advancing the DoD T&E Digital Engineering Ecosystem: Opportunities and Realities
The journey towards a mature Digital Engineering Ecosystem (DEE) for Department of Defense (DoD) Test and Evaluation (T&E) is characterized by significant opportunities for transformative improvements, alongside formidable realities in the form of technical, cultural, and organizational hurdles. Successfully navigating this landscape requires a clear understanding of the potential benefits, a candid assessment of the challenges, a commitment to workforce and cultural adaptation, and strategic actions to foster the ecosystem’s growth and effectiveness.
A. Quantifiable Benefits and Strategic Advantages for T&E
The adoption of Digital Engineering (DE) within the T&E domain promises a range of quantifiable benefits and strategic advantages that can significantly enhance the DoD’s ability to field effective and reliable weapon systems.
- Improved Efficiency and Cost Reduction: One of the most compelling arguments for DE is its potential to reduce the costs associated with traditional T&E. By leveraging models and simulations, the need for expensive physical mock-ups and extensive physical testing can be diminished.9 This can lead to overall lower T&E program costs, reduced risk of costly failures late in development, and better design quality achieved earlier in the lifecycle.10 The ability to shift defect detection to earlier phases through model-based analysis significantly reduces the high costs associated with rework that occur when problems are found later during physical testing or operational use.16
- Enhanced Speed and Agility: DE can dramatically accelerate T&E timelines and improve the responsiveness of the acquisition process. It fosters increased engagement between customers (warfighters) and vendors (developers), improves timelines for responding to evolving threats, and facilitates the rapid infusion of new technologies into systems.9 This ultimately contributes to a reduced time to market for critical capabilities.16 The use of digital tools enables rapid, data-driven decision-making, allowing T&E programs to adapt more quickly to changing requirements or emerging issues.17
- Improved Quality and Performance Insight: DE leads to more authoritative data management practices and supports decision-making that is better informed by rigorous modeling, simulation, and data analytics.1 This can result in higher quality systems with improved performance. The use of digital models allows for the exploration of more complex system designs and an expanded trade space for design alternatives during early acquisition phases, leading to more optimized solutions.1
- Better Risk Management: The ability to conduct virtual testing and analysis early in the lifecycle allows for earlier identification and mitigation of technical and programmatic risks.31 DE can also reduce uncertainty associated with system operations and sustainment by providing better predictive insights into long-term performance and reliability.1
- Enhanced Collaboration and Communication: Digital tools and common model-based environments improve collaboration and communication among diverse T&E stakeholders, including government teams, industry partners, and end-users.1 This shared understanding can lead to more effective decision-making and a more cohesive T&E effort.
While many of these benefits are widely claimed and intuitively understood, a critical step for the sustained advancement of the T&E DEE is the transition from anecdotal evidence to clearly demonstrable, measurable benefits. As noted in 1, “most benefits to date are anecdotal,” and there has been a lack of empirical analysis to robustly quantify the impact of DE. For continued investment and broad buy-in from leadership and practitioners alike, it is essential to establish clear metrics and systematically track the impact of DE on T&E outcomes. Examples of such metrics could include reductions in T&E cycle times, lower cost per test event or per hour of testing, improved defect discovery rates in earlier development phases (and correspondingly lower rates in later, more expensive phases), and increased test point coverage through the combined use of live and virtual testing. The observation that “Many survey respondents were not aware of how their leadership intends to measure DE success or what metrics are being tracked” 1 highlights a significant gap that must be addressed to build a compelling business case for DE in T&E and to guide its effective implementation.
Beyond these direct cost, schedule, and performance benefits, DE also offers significant second-order advantages related to the optimization of scarce and often expensive T&E resources. These resources include specialized test ranges, unique instrumentation, high-value test assets, and expert personnel. The current T&E landscape sometimes suffers from suboptimal resource allocation, potentially leading to “redundant or unnecessary testing”.11 By using M&S and other DE tools for more informed and comprehensive test planning, T&E programs can better explore the operational envelope virtually.3 This allows for the prioritization of live test events on the most critical and uncertain aspects of system performance, thereby making more efficient use of physical test resources and potentially reducing the overall burden on the T&E infrastructure.
B. Navigating the Challenges: Technical, Cultural, and Organizational Hurdles
Despite the compelling benefits, the path to a mature T&E DEE is fraught with significant challenges that span technical, cultural, workforce, cost, and governance domains.
- Technical Challenges: A primary and persistent technical hurdle is the lack of standardization and interoperability among the diverse array of tools, software packages, and models used within the DEE.1 This fragmentation can impede seamless data flow, create data silos, and necessitate costly and inefficient workarounds. Issues related to data availability, ensuring data quality, and managing vast quantities of data effectively are also prominent.1 The integration of modern DE practices with legacy T&E systems and data formats presents another complex technical problem.1 Furthermore, poorly performing or inadequate technical infrastructure can undermine DE efforts 1, and the inherent cybersecurity risks associated with managing large volumes of sensitive digital information must be rigorously addressed.9
- Cultural Challenges: Perhaps the most deeply entrenched challenges are cultural. There is often significant resistance to change, particularly in moving from long-established, document-based T&E processes to newer, model-based approaches.1 The traditional siloed operations of different T&E phases (e.g., CT, DT, OT) and disciplines (e.g., M&S developed separately from live test planning) also hinder the integrated vision of DE.11 Overcoming this inertia requires strong leadership, clear communication of benefits, and a willingness to adapt established norms.
- Workforce Challenges: The shift to DE necessitates a T&E workforce with new and evolving skill sets. There is a recognized shortage of personnel skilled in areas such as modeling and simulation, MBSE, data analytics, cybersecurity for digital environments, and the specific tools used in DE.1 Recruiting, training, and retaining a qualified workforce capable of operating effectively within the T&E DEE is a major undertaking.
- Cost Challenges: Implementing DE involves significant upfront investment costs. These include expenditures for IT infrastructure modernization, acquisition of new software licenses and modeling tools, development and maintenance of data management and governance systems, and the substantial costs associated with workforce training and development.1 Justifying these upfront costs can be difficult, especially when the full benefits may not be realized immediately.
- Governance and Intellectual Property (IP) Challenges: Establishing effective governance for critical components of the DEE, such as the Authoritative Source of Truth, is a complex task. This involves defining clear roles, responsibilities, data ownership, access controls, and quality standards.9 Additionally, protecting Intellectual Property rights for both government and industry partners, while simultaneously enabling the necessary collaboration and data sharing inherent in DE, presents a delicate balancing act.9
These challenges are often not isolated but are interconnected, creating a web of dependencies that can impede progress. For instance, a lack of tool interoperability (a technical challenge) can exacerbate workforce skill gaps, as personnel may need to become proficient in multiple, non-integrated toolsets. This, in turn, can fuel cultural resistance if digital workflows are perceived as cumbersome or inefficient due to these technical shortcomings. If the ASoT is difficult to populate or access due to tool issues or unclear data standards, trust in it will erode 9, making the cultural shift to model-based decision-making even harder.9 Addressing these intertwined challenges effectively requires a holistic, systems-thinking approach that considers these interdependencies rather than tackling each issue in isolation.
Furthermore, the difficulty of integrating or replacing legacy T&E systems and their associated data formats 1 represents a pervasive and significant drag on the speed and completeness of DE transformation within the T&E domain. Many existing T&E assets, including range instrumentation, data acquisition systems, and analytical tools, were developed long before the DE paradigm gained prominence. Replacing these systems wholesale is often financially prohibitive and programmatically disruptive. However, integrating them effectively into a modern DEE can be technically complex and may not always yield the full benefits of a natively digital approach. This reality means that DE adoption across the T&E enterprise might be uneven, with newer programs potentially embracing DE more fully and rapidly, while older systems and their supporting T&E infrastructure may lag, creating a mixed digital landscape for some time. This is not just a technical issue but also a significant budgetary and long-term planning challenge for the DoD.
Table 4: Major Challenges to Implementing DE in DoD T&E and Corresponding Mitigation Strategies
Challenge Category | Specific Challenge | Potential Impact on T&E DEE | Proposed Mitigation Strategy/Recommendation |
Technical | Lack of tool/model interoperability & standardization 1 | Data silos, inefficient workflows, manual data re-entry, limited Digital Thread effectiveness, increased training burden. | Mandate open standards, APIs, and common data formats for T&E tools; invest in developing and enforcing interoperability profiles; prioritize DoD DE Strategy focus on interfaces over specific tools.9 |
Data quality, availability, and management issues (not VAULTIS) 1 | Untrusted ASoT, flawed analyses, poor decision-making, inability to leverage advanced analytics/AI. | Implement robust data governance frameworks; invest in data curation and validation processes; adopt VAULTIS principles as a standard for T&E data.6 | |
Integration of legacy T&E systems and data 1 | Slows DE adoption, creates hybrid (digital/analog) workflows, limits end-to-end digital continuity. | Develop phased modernization plans for legacy T&E infrastructure; invest in middleware and data translators where full replacement is not feasible; prioritize DE for new systems. | |
Cybersecurity risks to models and data 9 | Compromise of sensitive T&E data, IP theft, corrupted models leading to incorrect evaluations. | Integrate cybersecurity into all phases of DE planning and execution; implement Zero Trust architectures 10; conduct regular vulnerability assessments of the DEE. | |
Cultural | Resistance to change from document-centric to model-based approaches 1 | Slow adoption of DE tools and processes, continued reliance on outdated methods, underutilization of DEE capabilities. | Strong leadership advocacy for DE; clear communication of benefits; pilot programs to demonstrate value; incentivize adoption of new practices; involve workforce in process redesign. |
Siloed operations (CT, DT, OT, M&S) 11 | Lack of integrated T&E, redundant efforts, missed opportunities for knowledge sharing, incomplete “whole body of evidence.” | Promote integrated T&E planning through T&E WIPTs; establish shared digital environments and ASoT; incentivize cross-functional collaboration. | |
Workforce | Shortage of skilled personnel (MBSE, M&S, data analytics) 1 | Inability to effectively use DE tools and methods, delays in DE implementation, reliance on external contractors. | Invest in comprehensive and continuous training programs; develop DE competency models for T&E 7; create career paths for DE T&E professionals; partner with academia. |
Difficulty retaining qualified DE workforce 1 | Loss of critical DE expertise, ongoing recruitment challenges, reduced return on training investment. | Offer competitive compensation and career advancement opportunities; foster a challenging and innovative work environment; provide access to modern tools and continuous learning. | |
Cost | High upfront investment for IT, tools, and training 1 | Budgetary constraints hindering DE adoption, perception of DE as an unfunded mandate. | Develop clear business cases demonstrating long-term ROI; advocate for dedicated DE funding; implement phased DE adoption with early, demonstrable wins to build momentum. |
Governance | Unclear ASoT data ownership and stewardship 9 | Confusion over data responsibility, potential for conflicting data sources, erosion of trust in the ASoT. | Establish clear ASoT governance charters defining roles, responsibilities, and decision rights for data domains; appoint data stewards for key T&E data. |
Protecting Intellectual Property (IP) while enabling collaboration 9 | Reluctance from industry to share detailed models/data; legal and contractual complexities. | Develop clear IP guidelines and contractual language for DE deliverables; implement secure collaboration environments with granular access controls; foster trust-based partnerships. |
C. Cultivating a Digitally Proficient T&E Workforce and Adaptive Culture
The successful maturation of the T&E DEE is inextricably linked to the cultivation of a digitally proficient workforce and an organizational culture that is adaptive and receptive to change. Technology alone is insufficient; it is the people who use the tools and execute the processes who will ultimately determine the success of this transformation.
The DoD Digital Engineering Strategy explicitly identifies the transformation of culture and workforce as one of its five strategic goals, emphasizing the need for focused efforts to lead and execute this change across the lifecycle.9 Similarly, the DOT&E Strategy Update 2022 dedicates its fifth pillar to “Foster an Agile and Enduring T&E Enterprise Workforce”.7 This pillar underscores the importance of identifying critical T&E workforce competencies, addressing professional development needs through continuous learning, and implementing robust recruitment and retention plans to attract and keep talent with digital skills.7
The shift towards DE demands a T&E workforce equipped with a new array of skills. Proficiency in areas such as systems modeling (using languages like SysML), advanced simulation techniques, data analytics, MBSE methodologies, cybersecurity principles relevant to digital environments, and the operation of specific DE software tools are becoming increasingly essential.1 This represents a significant departure from traditional T&E skill sets that may have been more focused on physical test execution and document-based analysis.
Beyond technical skills, a profound cultural shift is required. The T&E community must transition from its historically document-centric, often siloed operational modes to more collaborative, model-based, and data-driven approaches.1 This involves embracing transparency, sharing data and models more openly (within security constraints), and valuing early engagement in the development lifecycle.
Given the rapid and unceasing evolution of DE tools, platforms, and methodologies, particularly in fast-moving fields like AI/ML 11, efforts to cultivate a digitally proficient T&E workforce cannot be a one-time training initiative. Instead, they demand a sustained commitment to continuous learning, professional development, and adaptation. The DOT&E strategy’s call for an “effective continuous learning program” 7 directly acknowledges this reality. The T&E workforce must be equipped not only with the skills relevant to today’s digital tools and practices but, more importantly, with the foundational knowledge and mindset that enables them to learn, adapt, and leverage future digital innovations as they emerge throughout their careers and the long lifecycles of DoD systems.
While training programs and access to modern tools are vital components of workforce development, the transformation of the T&E organizational culture towards widespread DE adoption is primarily a leadership responsibility. Leaders at all levels within the T&E enterprise must actively champion the change. This involves clearly articulating the vision and strategic importance of DE, setting unambiguous expectations for its adoption, visibly using digital tools and data in their own decision-making processes, incentivizing new behaviors and skills, and consistently demonstrating the value that DE brings to the T&E mission and to the warfighter. The observation that early Army DE efforts were often “bold and independent initiatives without significant central control,” coupled with the acknowledgment of an eventual need for more centralized direction 2, suggests that while grassroots innovation is valuable, sustained, enterprise-wide cultural change requires concerted leadership. Without strong, consistent, and visible leadership commitment, cultural inertia and natural resistance to new ways of working 1 will likely prevail, hindering the full realization of the T&E DEE’s potential.
D. Strategic Recommendations for Maturing the Ecosystem
Maturing the DoD T&E DEE from its current state to a fully effective, enterprise-wide capability requires deliberate, strategic actions that address the identified challenges and build upon existing strengths. The following recommendations are proposed:
- Prioritize and Enforce Interoperability Standards: The DoD should accelerate the development, adoption, and enforcement of robust interoperability standards for T&E tools, models, and data. This includes mandating open architectures, common data formats (aligned with VAULTIS principles), and standardized Application Programming Interfaces (APIs) for all new T&E DEE components. A dedicated governance body should oversee compliance and the evolution of these standards.
- Invest in Sustained and Adaptive Workforce Development: Implement comprehensive, multi-tiered training and education programs focused on DE skills critical for T&E (MBSE, M&S, data science, AI/ML T&E, cybersecurity). These programs must be continuous and adaptive to keep pace with technological advancements. Develop clear career paths and certification programs for DE T&E professionals to incentivize skill acquisition and retention.
- Establish Clear DoD-Wide Governance for T&E ASoT: Develop and implement a clear, unambiguous governance framework for the T&E ASoT. This framework must define data ownership, stewardship responsibilities, access control policies, data quality standards, and configuration management procedures. It should also address complex issues of data rights and Intellectual Property management in collaborative environments involving industry partners.
- Develop and Mandate Standardized Metrics for DE Benefits in T&E: To move beyond anecdotal evidence, the DoD should develop and mandate a common set of metrics for measuring the impact and benefits of DE in T&E. These metrics should cover cost, schedule, performance, quality, and risk reduction. Regular reporting against these metrics will demonstrate value, justify investments, and identify areas for improvement.
- Fund Targeted Modernization of MRTFB Digital Infrastructure: Allocate dedicated funding for the targeted modernization of the Major Range Test and Facility Base (MRTFB) digital infrastructure. This includes upgrading range instrumentation, data acquisition systems, network connectivity, and local M&S capabilities, ensuring their seamless integration with the broader enterprise T&E DEE and ASoT.
- Foster Deeper Government-Industry Collaboration in DE for T&E: Establish more effective mechanisms for collaboration between government T&E organizations and their industry partners. This could include joint DE working groups, shared access to common digital environments (where appropriate and secure), and collaborative development of DE tools and best practices for T&E.
- Champion Cultural Change through Proactive Leadership: DoD leadership at all levels, particularly within the acquisition and T&E communities, must actively and visibly champion the cultural shift towards DE. This includes promoting a data-driven decision-making culture, rewarding innovation in DE application, and consistently communicating the strategic importance of the T&E DEE.
The maturation of the T&E DEE is not about optimizing individual components in isolation but requires the coordinated evolution of all its elements—stakeholders, processes, tools, infrastructure, and standards. These elements are deeply interdependent, and progress in one area can be hindered by deficiencies in another. For instance, investing heavily in advanced T&E tools like JSE will yield suboptimal results if the workforce is not adequately trained to use them effectively, or if data standards do not allow JSE to seamlessly interoperate with other critical systems like the ASoT or MBSE platforms. The very concept of an “ecosystem” implies this interconnectedness and co-dependence, necessitating a holistic approach to its development and sustainment.
Furthermore, while foundational policies like DoDI 5000.97 are crucial for setting direction and establishing mandates, their successful impact on the T&E DEE ultimately depends on robust implementation strategies, the allocation of dedicated resources, and effective mechanisms for enforcement and continuous improvement. The 2018 DE Strategy provided the “what,” and DoDI 5000.97 has provided more of the “how” 9, but the actualization of these directives across the diverse landscape of DoD T&E programs and organizations requires diligent oversight, performance monitoring (using the metrics recommended above), and a commitment to adapting strategies based on lessons learned and evolving technological opportunities. Policy alone is insufficient; sustained execution and a culture of continuous improvement are key to realizing the vision of a fully capable T&E DEE.
V. Conclusion: Charting the Future of DoD Test & Evaluation through Digital Engineering
The adoption of Digital Engineering represents a pivotal transformation for the Department of Defense, and its application within the Test and Evaluation domain is critical to realizing the overarching goals of accelerated capability delivery, enhanced system performance, and sustained technological advantage. The T&E Digital Engineering Ecosystem—a complex interplay of stakeholders, digitally transformed processes, advanced tools and platforms, robust supporting infrastructure, and governing standards—is the essential foundation upon which this transformation is built.
This analysis has detailed the multifaceted nature of the T&E DEE, emphasizing how core enablers such as the Authoritative Source of Truth, digital models, digital twins, the digital thread, Model-Based Systems Engineering, and Artificial Intelligence are fundamentally reshaping the T&E paradigm. The potential benefits are profound, offering pathways to greater efficiency, reduced timelines, lower costs, improved system quality, and more insightful risk management. However, the journey is not without significant challenges, including technical interoperability hurdles, the need for substantial cultural and workforce adaptation, and the complexities of governance in a digital environment.
The interconnectedness of the ecosystem’s components cannot be overstated. Progress in maturing the T&E DEE requires a holistic and coordinated approach, recognizing that advancements in one area are often contingent upon progress in others. This is not a one-time technological upgrade but an ongoing journey of transformation that demands sustained commitment, strategic investment, agile adaptation to new technologies and methodologies, and unwavering leadership.
Looking forward, a mature and fully realized T&E Digital Engineering Ecosystem will empower the DoD to conduct more comprehensive, insightful, and timely evaluations of increasingly complex warfighting systems. It will enable a shift from periodic, often isolated test events to a continuous, data-driven evaluation process that spans the entire system lifecycle. Ultimately, by embracing and advancing the T&E DEE, the Department of Defense will be better positioned to deliver superior, resilient, and adaptable capabilities to the warfighter at the speed of relevance, ensuring mission success in the dynamic and contested operational environments of the future.
VI. Glossary of Key Acronyms and Terms
- AI/ML: Artificial Intelligence / Machine Learning
- ASoT: Authoritative Source of Truth
- ATEC: Army Test and Evaluation Command
- AFOTEC: Air Force Operational Test and Evaluation Center
- CDT: Chief Developmental Tester
- CI/CD: Continuous Integration / Continuous Delivery
- COCOM: Combatant Commander
- CODEX: Coverage of Data Explorer
- CT: Contractor Testing
- DE: Digital Engineering
- DEE: Digital Engineering Ecosystem
- DEF: Developmental Evaluation Framework
- DIB: Defense Industrial Base
- DoD: Department of Defense
- DoDI: Department of Defense Instruction
- DOT&E: Director, Operational Test and Evaluation
- DT: Developmental Testing
- FFRDC: Federally Funded Research and Development Center
- HPC: High-Performance Computing
- IC: Intelligence Community
- IDSK: Integrated Decision Support Key
- IP: Intellectual Property
- ISO: International Organization for Standardization
- IT: Information Technology
- JATIC: Joint AI Test Infrastructure Capability
- JITC: Joint Interoperability Test Command
- JMETC: Joint Mission Environment Test Capability
- JSE: Joint Simulation Environment
- LFT&E: Live Fire Test and Evaluation
- M&S: Modeling and Simulation
- MBSE: Model-Based Systems Engineering
- MB-TEMP: Model-Based Test and Evaluation Master Plan
- MCOTEA: Marine Corps Operational Test and Evaluation Activity
- MRTFB: Major Range Test and Facility Base
- ONNX: Open Neural Network Exchange
- OPTEVFOR: Operational Test and Evaluation Force (U.S. Navy)
- OT: Operational Testing
- OUSD(R&E): Office of the Under Secretary of Defense for Research and Engineering
- PEO: Program Executive Office
- PLM: Product Lifecycle Management
- PM: Program Manager
- QMS: Quality Management System
- SERC: Systems Engineering Research Center
- SoR: System of Record
- STPA: Systems Theoretic Process Analysis
- SysML: Systems Modeling Language
- T&E: Test and Evaluation
- TEMP: Test and Evaluation Master Plan
- TENA: Test and Training Enabling Architecture
- TRMC: Test Resource Management Center
- V&V: Verification and Validation
- VAULTIS: Visible, Accessible, Understandable, Linked, Trustworthy, Interoperable, Secure
- WIPT: Working Integrated Product Team
VII. References
1
Works cited
- www.rand.org, accessed May 21, 2025, https://www.rand.org/content/dam/rand/pubs/research_reports/RRA2300/RRA2333-2/RAND_RRA2333-2.pdf
- An Independent Assessment of the Army Implementation of Digital Engineering (DE), accessed May 21, 2025, https://asb.army.mil/Portals/105/Reports/2020s/2023%20Digital%20Engineering.pdf?ver=iE2ADXCDoajwLvNWthpHnw%3D%3D
- Digital Engineering, Modeling and Simulation – DoD Research & Engineering, OUSD(R&E), accessed May 21, 2025, https://www.cto.mil/sea/dems/
- Digital Engineering Ecosystem | www.dau.edu, accessed May 21, 2025, https://www.dau.edu/glossary/digital-engineering-ecosystem
- www.cto.mil, accessed May 21, 2025, https://www.cto.mil/wp-content/uploads/2024/01/info-dodi-500097-de-summary.pdf
- The DoD’s New Digital Engineering Directive’s Impact on Work …, accessed May 21, 2025, https://www.canvasgfx.com/blog/dod-digital-engineering-directive
- DOT&E Strategy Implementation Plan – 2023 – Director Operational …, accessed May 21, 2025, https://www.dote.osd.mil/Portals/97/pub/reports/DOTE_Strategy_Imp_Plan-Apr2023.pdf
- The Department of Defense Announces its Digital Engineering Strategy, accessed May 21, 2025, https://www.defense.gov/News/Releases/Release/Article/1567723/the-department-of-defense-announces-its-digital-engineering-strategy/
- man.fas.org, accessed May 21, 2025, https://man.fas.org/eprint/digeng-2018.pdf
- Digital Engineering Is Growing in Federal Organizations, Fueled by Quality and Speed, accessed May 21, 2025, https://www.meritalk.com/articles/digital-engineering-is-growing-in-federal-organizations-fueled-by-quality-and-speed/
- Digital Engineering for Test and Evaluation, accessed May 21, 2025, https://sercuarc.org/wp-content/uploads/2024/11/100pm_SRR-2024-Freeman-Digital-Trans.pdf
- Knowledge Based Metrics for Test and Design – Excerpt from the Proceedings – Naval Postgraduate School, accessed May 21, 2025, https://dair.nps.edu/bitstream/123456789/5348/4/SYM-AM-25-314.pdf
- Excerpt from the Proceedings – DAIR – Acquisition Research Program, accessed May 21, 2025, https://dair.nps.edu/bitstream/123456789/5412/1/SYM-AM-25-360.pdf
- Digital Twin: A Quick Overview – International Test and Evaluation Association, accessed May 21, 2025, https://itea.org/journals/volume-45-1/digital-twin-a-quick-overview/
- Model-Based TEMP Strategy & Integrated Decision Support Key – The Acquisition Innovation Research Center, accessed May 21, 2025, https://document.acqirc.org/publication_documents/reports/Model-Based%20TEMP%20Workshop%20Summary_Final.pdf
- itea.org, accessed May 21, 2025, https://itea.org/wp-content/uploads/2020/12/MBSE-for-Test-_HONEA-ITEA-SYMP-2020-Final.pdf
- How Digital Twins and Digital Engineering Drive Innovation and …, accessed May 21, 2025, https://governmenttechnologyinsider.com/how-digital-twins-and-digital-engineering-drive-innovation-and-efficiency-in-dod-systems/
- MBSE Wiki – Authoritative Source of Truth, accessed May 21, 2025, https://www.omgwiki.org/MBSE/doku.php?id=mbse:authoritative_source_of_truth
- Digital Engineering for Defense and Intelligence Mission Success | OD42 – YouTube, accessed May 21, 2025, https://www.youtube.com/watch?v=Vrq-eDXr6N4
- Test Resource Management Center – Office of the Under Secretary of Defense for Research and Engineering, accessed May 21, 2025, https://www.cto.mil/trmc/
- Test Resource Management Center, accessed May 21, 2025, https://www.trmc.osd.mil/
- Test & Evaluation Master Plan (TEMP) | www.dau.edu, accessed May 21, 2025, https://www.dau.edu/acquipedia-article/test-evaluation-master-plan-temp
- Overview of DoD Modern Software T&E, Adapting Software Developmental Test Guidance – DAU, accessed May 21, 2025, https://www.dau.edu/sites/default/files/2025-02/SW%20DTE%20DSO%20Guidebook%20Engagement.pdf
- Test and evaluation master plan – Wikipedia, accessed May 21, 2025, https://en.wikipedia.org/wiki/Test_and_evaluation_master_plan
- Modeling and simulation – Exploring lethality before it’s built – Wright-Patterson AFB, accessed May 21, 2025, https://www.wpafb.af.mil/News/Article-Display/Article/4177397/modeling-and-simulation-exploring-lethality-before-its-built/
- Joint Simulation Environment Overview – NAVAIR, accessed May 21, 2025, https://www.navair.navy.mil/nawcad/sites/g/files/jejdrs546/files/document/%5Bfilename%5D/Joint%20Simulation%20Environment%20Overview_PAO2023-0057_0.pdf
- Analytic Tools – Chief Digital and Artificial Intelligence Office, accessed May 21, 2025, https://www.ai.mil/Initiatives/Analytic-Tools/
- Digital Engineering Instruction (DoDI 5000.97) Definition – Arena Solutions, accessed May 21, 2025, https://www.arenasolutions.com/resources/glossary/digital-engineering-instruction/
- MBSE Wiki – Digital Engineering Ecosystem, accessed May 21, 2025, https://www.omgwiki.org/MBSE/doku.php?id=mbse:digital_engineering_ecosystem
- Authoritative Source of Truth | www.dau.edu, accessed May 21, 2025, https://www.dau.edu/glossary/authoritative-source-truth
- Model Based Systems Engineering (MBSE), accessed May 21, 2025, https://www.baesystems.com/en-us/our-company/inc-businesses/intelligence-and-security/capabilities-and-services/mbse